Discussion
Discussion
For each training dataset, i.e. (Country, Rap), (Country, Jazz), (Rap, Jazz) and (Country, Rap, Jazz), we extracted the top 50 frequently-appeared words of each genre. The score of each word is obtained by adding up the TFIDF value of the word that appeared in each song in that specific genre. For each training dataset, we compared their 50 frequently-appeared words, extracting the words in common and the words that are different in each genre.
Words that are extracted from top 50 frequently-appeared words in two genres:
Country, Rap
Country, Jazz
Rap, Jazz
Country, Rap, Jazz
From the scores we obtained for each word, we observed that the top frequently appeared words (word with highest scores) in each dataset are mostly words in common (words that appeared in each genre).
However, from the histogram of common words, we can see that in general, the frequently appeared common words have similar score in each genre, and the histogram follows pretty similar trend. This indicates that these frequently appeared common words are not the main features for each genre and therefore are not the main reason for classifiers to perform different classifications.
As for frequently appeared words that are not in common, we first take a look at (Country, Rap) dataset. The scores of words in Country but not in Rap, and the scores of words in Rap but not in Country are both high. Top score 138 for “up” in Rap and top score 148 for “love” in Country. We think that the high scores (refer to high TFIDF weight) of words not in common in each genre makes (Country, Rap) dataset easily distinguishable. Therefore result in the relatively high accuracy when classifying this dataset.
For (Country, Jazz) dataset, we also observe frequently appeared words that are not in common for each genre.The top scores of words in Country but not in Jazz is 107, and the top scores of words in Jazz but not in Country is 79. Compared to the highest score in the dataset (>200), the scores are relatively low. We think that this could be one of the reason that makes the classifiers harder to classify this dataset. The accuracy for classifying this dataset is relatively low.
For (Rap, Jazz) dataset, we again observe frequently appeared words that are not in common for each genre.The top scores of words in Rap but not in Jazz is 151 for the word ”up”, and the top scores of words in Jazz but not in Rap is 193 for the word “love”. Again, both scores are high. This makes the dataset easily distinguishable and results in relatively high accuracy when classifying this dataset.
For (Country, Rap, Jazz) dataset, the top scores of words in Rap but not in Jazz and Country is 148 for the word ”get”, the top scores of words in Country but not in Rap and Jazz is 93.9 for the word “her”, and the top scores of words in Jazz but not in Rap and Country is 78.8 for the word “will”. The top scores of words in Rap but not in Jazz and Country is relatively high. We printed out the error classification of test data and find out most error predictions occur due to the classifier misclassify Country music to Jazz genre or Jazz music to Country. This agree with our expectation, that Rap is the most distinctive type in this dataset and is the easiest distinguishable one.
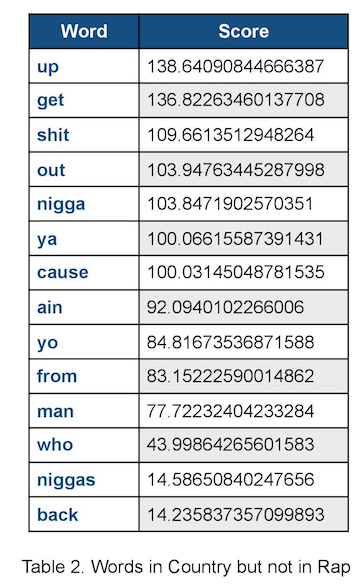


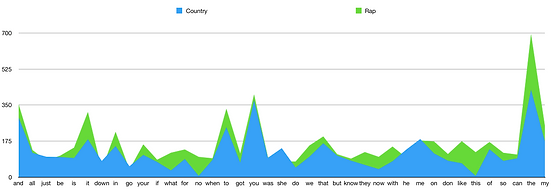


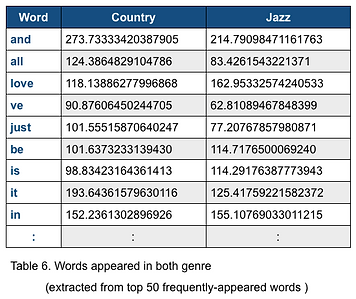

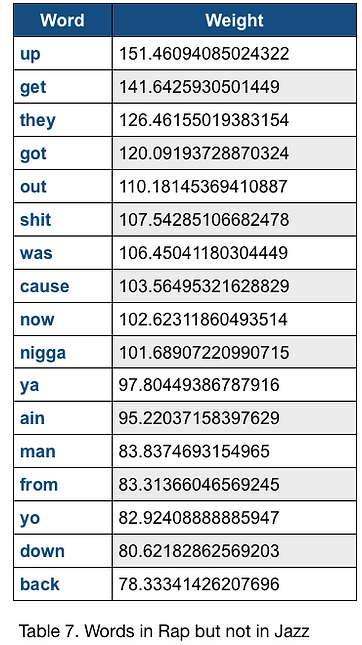
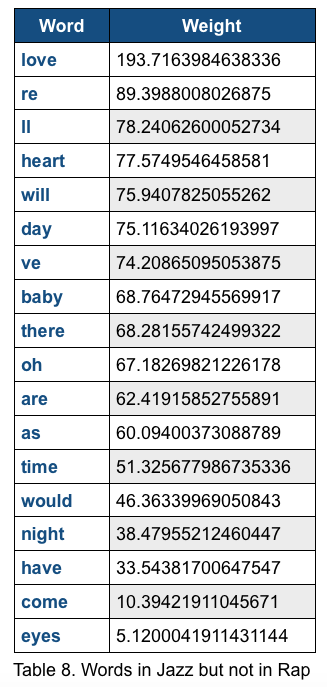


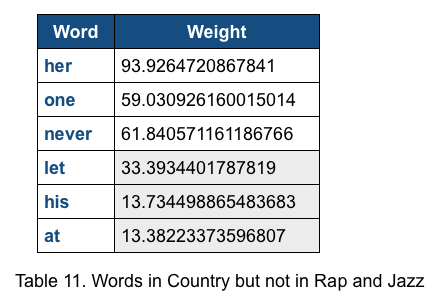
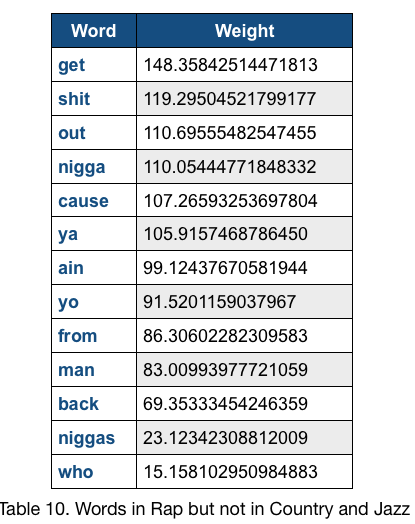
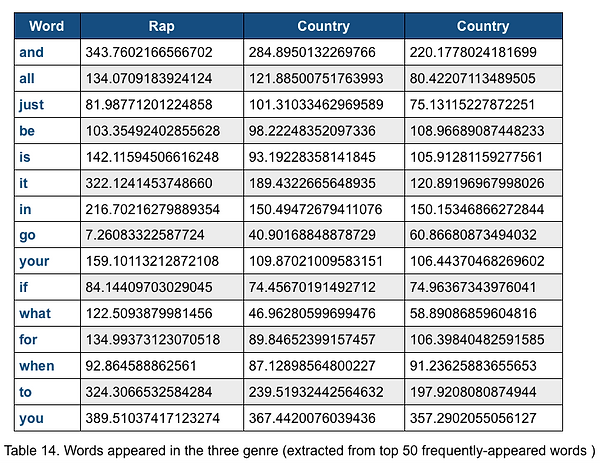
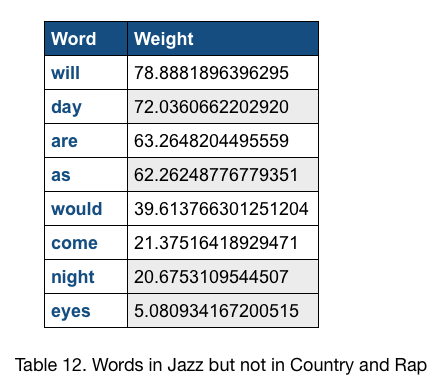
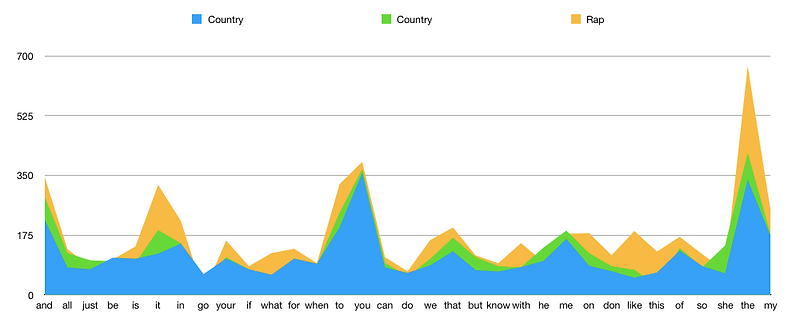
Figure 2. The histogram of common words in Country and Jazz
Figure 3. The histogram of common words in Jazz and Rap
Figure 4. The histogram of common words in Country, Rap and Jazz
Table 2. Words in Rap but not in Country